These white papers offer new perspectives
The force behind RESOLV is Dr. David Groeneveld, whose interest in satellite imaging traces back to the inception of the first Landsat program in the mid-1970s. David’s innovative applications for Earth observation reflectance data have resulted in high-profile groundbreaking projects: expert testimony in state and federal water rights cases, multiple regional water resource assessments and models, a precision irrigation application sold to Monsanto/Climate Corp, an ecological model to predict vegetation responses for Sandia Labs and U.S. DOE, authorship and negotiation for provisions that settled an 85-year-old water resources vs. environment controversy (Los Angeles and Owens Valley, CA), and a satellite-based monitoring program for dust control measures for the City of Los Angeles and the managing Air Pollution Control District, now in use for over two decades.
David’s mission is now RESOLV customer service and the RESOLV “Next-gen” program to accurize and standardize RESOLV calibration and application to any EO sensor operating in the VNIR spectral range.
CMAC versus Sen2Cor Software Surface Reflectance from Sentinel-2 Data
Note: The algorithm built into the RESOLV service is CMAC (Closed-form Method for Atmospheric Correction). CMAC has been used in our publications and is also used throughout these white papers.

We summarize our recent journal paper here introducing CMAC software and comparing it to Sen2Cor for atmospheric correction of Sentinel-2 data. Similarly, a topic paper comparing CMAC to LaSRC software of Landsat applies the same methods. Sen2Cor and LaSRC are industry standards responsible for virtually all surface reflectance processing, either directly or through transference to smallsats.
CMAC versus LaSRC Software
for Landsat Data

This paper recaps analyses and results in our second journal paper that compared CMAC and LaSRC software performance for atmospheric correction of Landsat 8 and 9 images. The methods were the same as used in the white paper evaluation of Sen2Cor (above). Like Sentinel 2a and 2b, the L8 and L9 sensor responses are virtually identical, so were not differentiated in this analysis. LaSRC corrected images were downloaded from Earth Explorer.
CMAC applies a completely different workflow than Sen2Cor and LaSRC. With no common methodology touchpoints, CMAC is best evaluated through comparative performance with existing methods. CMAC and LaSRC results agreed closely for images obtained through relatively clean atmospheres. LaSRC Accuracy was severely impacted for images obtained for moderate to extreme levels of wildfire smoke while CMAC provided accurate surface reflectance retrieval in all cases.
Reliability and Stability of CMAC Compared to LaSRC

As a first-order approximation, color balance and clarity can verify that atmospheric correction results are close to true surface reflectance. If so, the resulting image will be clear of haze and present natural appearing colors. Such comparisons between CMAC and LaSRC have repeatedly shown wide differences that would hypothetically result from systematic divergence of surface reflectance estimates. This was investigated for atmospherically corrected Landsat 8 data is the region near Lake Newell, Alberta, Canada. The disparity between the color balance of the CMAC and LaSRC corrections was determined to result from changes in statistical properties for targets known to remain consistent through the period of study. Although the CMAC version looks appropriate, the question was asked, which is correct? This question was examined using reflectance distributions between high and low spectral diversity AOIs.
This paper predated the third Juried Paper (links in Peer Reviewed Papers page) and prompted additional analyses that that confirmed that LaSRC output for low dark to bright spectral diversity is unreliable. CMAC output remained stable and correct despite aerosol loading. Sen2Cor has not been tested, but extensive observations of many image corrections has indicated that Sen2Cor shares this LaSRC limitation.
Mapping Atmospheric Effect Grayscales with Scene Statistics
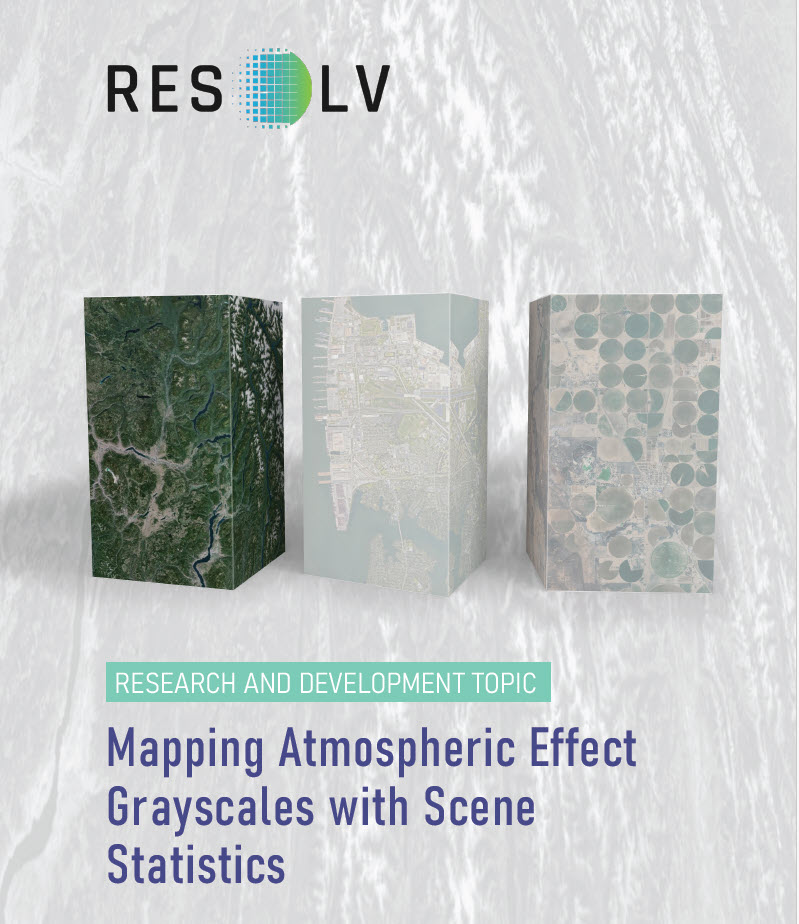
As a first-order approximation, color balance and clarity can verify that atmospheric correction results are close to true surface reflectance. If so, the resulting image will be clear of haze and present natural appearing colors. Such comparisons between CMAC and LaSRC have repeatedly shown wide differences that would hypothetically result from systematic divergence of surface reflectance estimates. This was investigated for atmospherically corrected Landsat 8 data is the region near Lake Newell, Alberta, Canada. The disparity between the color balance of the CMAC and LaSRC corrections was determined to result from changes in statistical properties for targets known to remain consistent through the period of study. Although the CMAC version looks appropriate, the question was asked, which is correct? This question was examined using reflectance distributions between high and low spectral diversity AOIs.
This paper predated the third Juried Paper (links in Peer Reviewed Papers page) and prompted additional analyses that that confirmed that LaSRC output for low dark to bright spectral diversity is unreliable. CMAC output remained stable and correct despite aerosol loading. Sen2Cor has not been tested, but extensive observations of many image corrections has indicated that Sen2Cor shares this LaSRC limitation.
Reversing the Atmospheric Effect Mapped by the Atm-I Grayscale
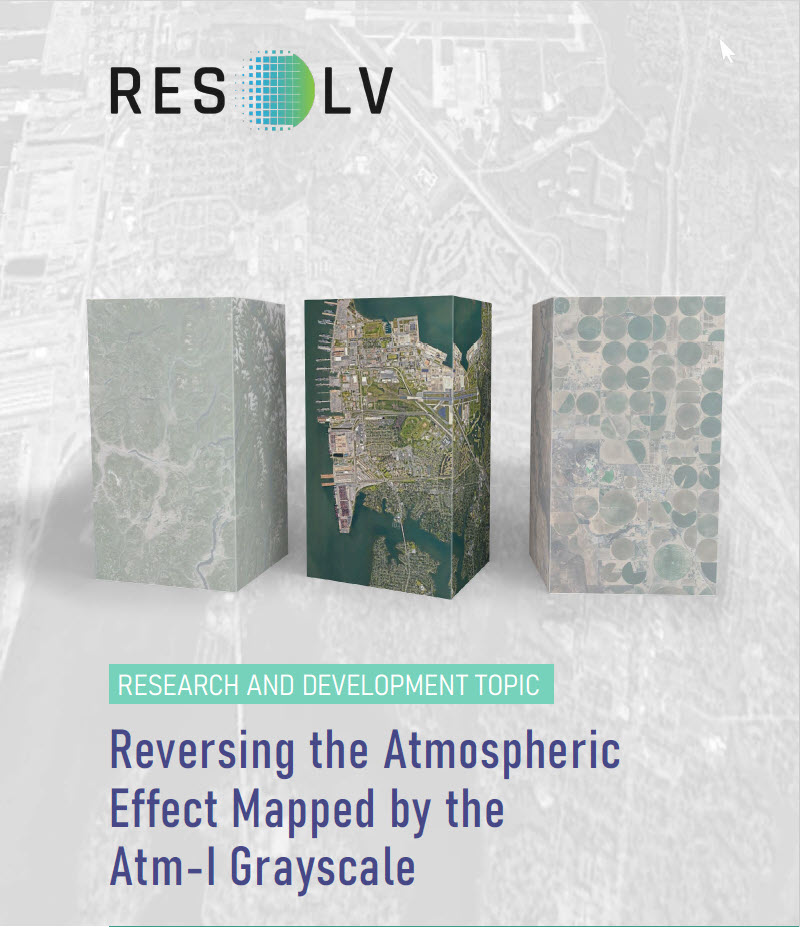
CMAC development began with a unique discovery: changes in cumulative distribution functions (CDFs) for top-of-atmosphere reflectance (TOAR) occur in a structured pattern. Comparing clear and hazy images for the same area of interest (AOI) across short time spans shows that increasing haze causes reflectance CDFs to rotate counterclockwise, and for decreasing haze, to rotate clockwise. We called this the “pinwheel effect” due to the rotation of the CDF from the changing atmospheric effects. The pinwheel effect was translated into a conceptual model by inverting and adjusting the axes of the well-known empirical line method. The resulting conceptual model has precedence in a prestigious remote sensing paper from 1985.
This CMAC conceptual model summarizes atmospheric effect as a linear relationship that we dubbed the “TOAR deviation line” that encodes the change of reflectance from SR to TOAR. Each TOAR deviation line represents all pixel reflectances, dark to bright, lying under the same airmass that is represented by the atmospheric index, Atm-I. This line is uniquely defined by its slope and y-intercept. The slope and intercept are calibrated to Atm-I to provide curves for translating the Atm-I grayscale into the required slopes and offsets. These parameters allow reversing the TOAR to surface reflectance spatially across images.
Forward Scatter and Its Effects on Atmospheric Correction
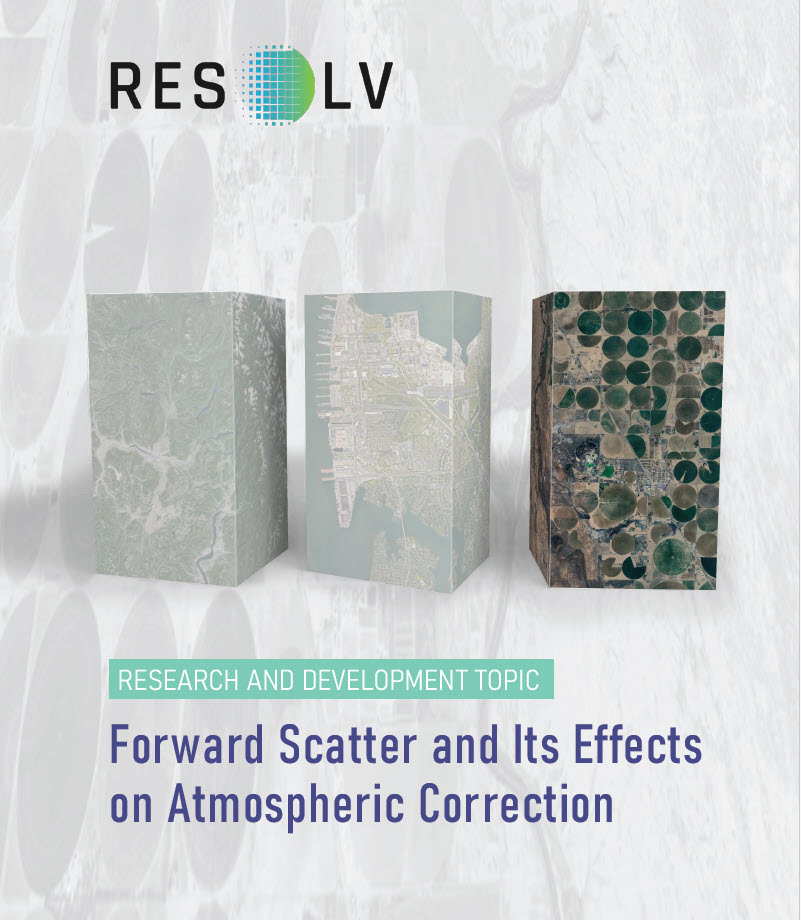
A first indication of forward scatter was observed while we were developing the Atm-I model. The resulting grayscale output indicated locations across images that were affected by the atmosphere to a greater degree than the surrounding area. Bright rooftops, rock outcrops, bare soil and specular reflectance off of water bodies were portrayed much brighter in the Atm-I grayscale than would be expected were backscatter from atmospheric particles the sole mechanism at work. Another indication of forward scatter that can be readily observed in TOAR data is that bright targets tend to expand to neighboring pixels while dark targets contract – this is illustrated for same sized black and white panels of targets arrayed in the typical checkerboard pattern. This phenomenon tends to limit the quality of atmospheric correction as Atm-I increases and greatly affects bright targets
From our relatively cursory familiarity with remote sensing literature, important role played by forward scatter in atmospheric correction has not been recognized in the literature.
NDVI from Atmospherically Corrected Satellite Data
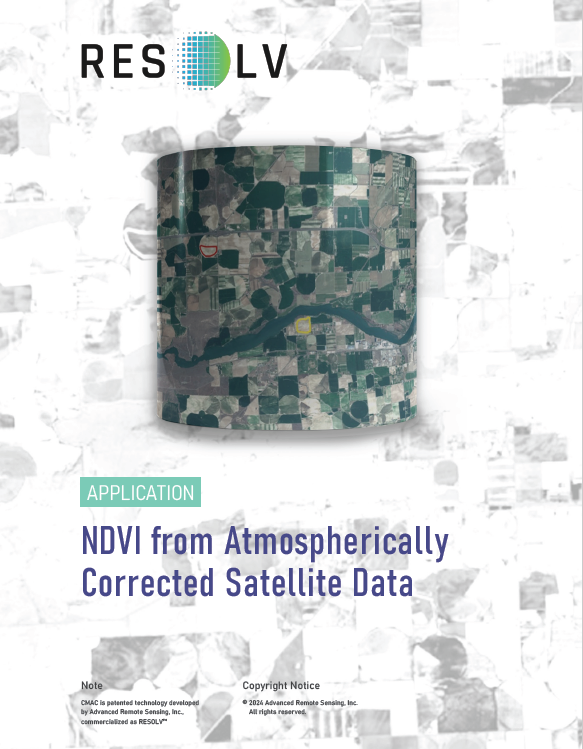
NDVI (normalized difference vegetation index) and similar indices represent plant activity mathematically to express vigor that directly translates to photosynthetic production, carbon uptake, crop yield, etc. In agriculture, NDVI is the most commonly used of many indices because it is both simple and reliable: NDVI = (NIR – Red) / (NIR + Red). Nearly all vegetation indices apply red and near infrared (NIR) bands to evaluate plant vigor.
NDVI can be used to evaluate the quality of atmospheric correction when displayed in an image time series. True plant growth curves change through time smoothly, without jagged up and down diversions induced by variable atmospheric effect. This fact was used to evaluate the accuracy of corrected data though a time series display of multiple S2 images. NDVI from CMAC correction accurately portrayed crops from germination at the beginning to senescence at the season’s end. In contrast, both TOAR and Sen2Cor NDVI were negatively influenced by atmospheric conditions. A method for indexing crop growth afforded by the accuracy and stability of the CMAC NDVI-time-series is demonstrated that renders growing degree day calculations obsolete. This satellite data indexing method is applicable under automation across the vast scale of satellite imagery.
In our commitment to excellence, RESOLV’s method has undergone rigorous comparative analysis against established atmospheric correction software. RESOLV has been benchmarked against LaSRC and Sen2Cor and has successfully been applied to high-resolution smallsat data.
RESOLV’s completely new approach starts by mapping the atmospheric effect across each image as a grayscale input to an algorithm that is based on observations of the behavior of light after transmission through Earth’s atmosphere. This algorithm inverts and adjusts the accepted empirical line method resulting in a closed-form equation that provides lightning-fast calculations. Through this structure, RESOLV delivers the most accurate estimates of surface reflectance possible for any image anywhere.
Key findings from the comparison include:
- Proven Accuracy: RESOLV consistently delivers high levels of accuracy in surface reflectance data, often surpassing other methods in challenging atmospheric conditions.
- Designed Efficiency: RESOLV’s intricate design ensures rapid data processing, delivering near real-time surface reflectance data of impeccable quality.
- Future-Ready Versatility: Beyond mere proof-of-concept, RESOLV has exhibited its adaptability, seamlessly integrating with a diverse range of smallsats.
Join us as we explore atmospheric correction of satellite images and how this unlocks the data for precision applications.
In our commitment to excellence, RESOLV’s method has undergone rigorous comparative analysis against established atmospheric correction software. RESOLV has been benchmarked against LaSRC and Sen2Cor and has successfully been applied to high-resolution smallsat data.
RESOLV’s completely new approach starts by mapping the atmospheric effect across each image as a grayscale input to an algorithm that is based on observations of the behavior of light after transmission through Earth’s atmosphere. This algorithm inverts and adjusts the accepted empirical line method resulting in a closed-form equation that provides lightning-fast calculations. Through this structure, RESOLV delivers the most accurate estimates of surface reflectance possible for any image anywhere.
Key findings from the comparison include:
- Proven Accuracy: RESOLV consistently delivers high levels of accuracy in surface reflectance data, often surpassing other methods in challenging atmospheric conditions.
- Designed Efficiency: RESOLV’s intricate design ensures rapid data processing, delivering near real-time surface reflectance data of impeccable quality.
- Future-Ready Versatility: Beyond mere proof-of-concept, RESOLV has exhibited its adaptability, seamlessly integrating with a diverse range of smallsats.